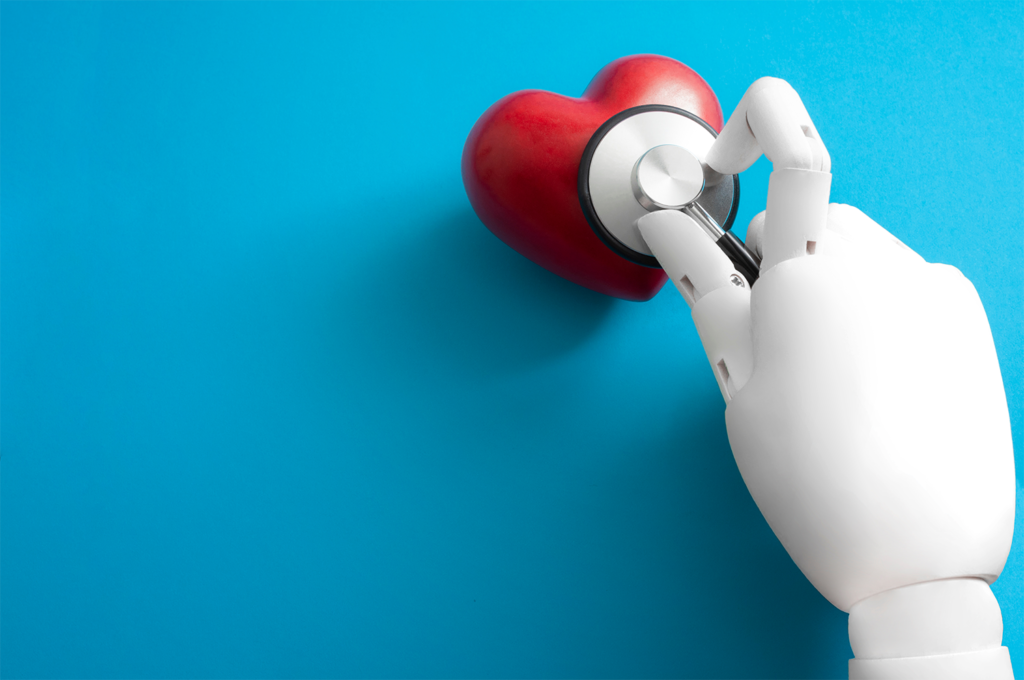
A question of how, not if
Artificial intelligence (AI) in healthcare is already a reality.[1] Healthcare providers have embedded the technology into their workflows and the decision-making processes. The introduction of AI in healthcare has brought improvements for patients, providers, payers and other healthcare stakeholders, as well as society at large. However, the European Coordination Committee of the Radiological, Electromedical and Healthcare IT Industry (COCIR) says there are key challenges that must be dealt with for the full benefits of AI in healthcare to be realised.[2]
The foundational concepts of AI were laid more than fifty years ago. However, it was only relatively recently that the twin effects of an exponential increase in computational power coupled with the omnipresence of data made AI a powerful, practical reality.
The introduction of AI into healthcare has brought improvements at various levels. For example, on the patient level, it allows for more accurate and/or rapid detection, diagnosis and treatment, resulting in improved outcomes.
However, the full benefits of AI in healthcare will only be realised if the key challenges we currently face in the fields of access to data; go-to-market; regulatory and technical matters; legal matters; and the ethical framework are appropriately identified and addressed.
Access to data
The efficacy of AI applications relies heavily on access to datasets on which the system has been trained. The higher the quality of data that goes into the system, the better the outcome of the AI-specific task. Without unhindered access to high quality data at scale, the huge potential of AI will not be realised in healthcare.
On the one hand, it is a matter of quantity: the more data available, the larger the datapool and sample patient group(s) on which the system can be trained to detect patterns. On the other hand, and even more importantly, there is a need for high quality data. Although data is all around us, it can be challenging to get access to material that meets the required standard.
Data that is available may require additional curation before it can be put to good use, for instance, by cleaning or labelling it, or linking several data repositories together in relation to a single patient. Furthermore, it is important to identify whether the available data sets may create or strengthen any bias in the outcomes.
To ensure a level playing field and spur innovation, it is vital that access to high quality data is fair, transparent and non-discriminatory.
Go-to-market
The various possible AI applications, methodologies, use cases and so on that are either already on the market, or will be in the future, may have different go-to-market plans lined up. This section outlines a couple of the most reasonable scenarios in a non-exhaustive list.
Healthcare providers are interested in supportive tools to improve their decision-making for diagnostic and therapeutic procedures, or any clinical decision at the point of care.
While there are already useful stand-alone algorithms in clinical use for medical image interpretation, AI’s broader use in daily healthcare requires versatile platforms to support procedures and decision-making in a streamlined way, so that all necessary applications can be easily integrated into existing workflows and information technology (IT) infrastructure.
Traditionally, AI algorithms are developed to solve a well-defined clinical problem. However, in reality, healthcare is much more complex—e.g. reading a chest CT scan and distinguishing between emphysema and lung nodes, coronary arteries, the aorta, the vertebrae—so multiple algorithms need to work together to best support decision-making.
The go-to-market and deployment scenarios, and by extension the adoption of AI in healthcare, will not so much depend on the question of whether or not to use the software, but more on how to integrate different applications into existing infrastructures and physician workflows.
Examples of such scenarios might be offering AI solutions embedded in a picture archive and communication system (PACS) reading workstation in a radiology department, or deploying them through some form of app store where healthcare providers can access a wide collection of applications and algorithms.
Each vendor will need to carefully consider the best way of deployment to fulfill the clinical needs of healthcare providers. Many different scenarios can apply, ranging from smaller vendors focussing on single-use applications to bigger vendors offering AI platforms, assistance-like systems or online marketplaces.
In this respect, it is also crucial to consider the appropriate business model. Traditionally, the main business model was to still sell licences and support services. However, other options are being explored and introduced, one of the most prominent being subscription or volume-based Software-as-a-Service (SaaS) models. These models are also increasingly used for AI applications.
While these business models run as ‘transactional models’ and can be performed between all market participants (providers, payers, vendors and others), it is mission-critical that there is a transparent and robust ‘order to cash’ process, which can also be audited and prove accuracy in billing of the transactional volume.
Regulatory and technical matters
To a large extent, AI may be simply considered as a specific type of software. The general safety and performance requirements are generic principles, which do not necessarily require adaptation to a new technology, hence existing regulations should be applied.
As different AI approaches, such as machine learning and deep learning, may be applied by different industries, current standardisation work ongoing within various standardisation bodies aims to establish good practices. Such standards should be endorsed to create a reliable pathway to market for manufacturers, notified bodies and regulators alike.
Legal matters
AI does not operate in a vacuum. Any deployment in the field will trigger a number of legal obligations under existing frameworks and may raise a number of critical questions.
There is, for instance, the matter of liability. In this respect, a distinction can be made between applications that support decision-making and those that can make autonomous decisions (e.g. autonomous driving).
The healthcare industry is offering AI applications in support of clinical decision-making; for instance, by highlighting suspicious regions in an image. However, the physician performs any downstream decision to further manage patients, and therefore the liability lies with the user/physician.
Liability needs to be considered in various scenarios, such as unforeseen use, off-label use, user errors, inadequate training, lack of maintenance or defective products.
The development of AI may also raise a number of questions regarding intellectual property rights(IPR). In cases where AI algorithms are being built in close collaboration with clinical partners, questions referring to IPR need to be addressed in advance.
Ethical framework
On 25 April 2018, the European Commission announced its Strategy on Artificial Intelligence, one of the main pillars of which is to ensure an appropriate ethical and legal framework.[3] Whereas much power has been attributed to AI, the algorithms that run these systems are developed by humans. Consequently, any bias or ethical considerations that consciously or unconsciously are programmed into the system will determine the output of these AI systems.
The Ethics Guidelines for Trustworthy AI were published by the European Commission on 8th April 2019.[4] They list seven key requirements that AI systems should meet in order to be deemed trustworthy:
- human agency and oversight;
- technical robustness and safety;
- privacy and data governance;
- transparency;
- diversity, non-discrimination and fairness;
- societal and environmental well-being; and
- accountability.
The Ethics Guidelines present an assessment list that offers guidance on each requirement’s practical implementation. This assessment list will undergo a piloting process in order to gather feedback for its improvement.
Conclusion
It is clear that AI in healthcare has great potential, and the best possible conditions should be created to enable further growth and expansion into uncharted healthcare territories, bringing benefits to patients, physicians and healthcare providers, as well as society at large.
COCIR is the European Trade Association representing the medical imaging, radiotherapy, health information and communications technology, and electromedical industries. Founded in 1959, COCIR is a non-profit association headquartered in Brussels with a China Desk based in Beijing since 2007. COCIR is unique as it brings together the healthcare, IT and telecommunications industries. Our focus is to open markets for COCIR members in Europe and beyond. We provide a wide range of services on regulatory, technical, market intelligence, environmental, standardisation, international and legal affairs. COCIR is also a founding member of DITTA, the Global Diagnostic Imaging, Healthcare IT and Radiation Therapy Trade Association (globalditta.org).
[1] A library of use cases will be published on the COCIR website, http://www.cocir.org/
[2] This article is an abridgement of COCIR’s White Paper on Artificial Intelligence in Healthcare, released in April 2019, which can be downloaded from the COCIR website.
[3] Artificial Intelligence for Europe, European Commission, 25th April 2018, viewed 6th May 2020, <http://ec.europa.eu/newsroom/dae/document.cfm?doc_id=51625>
[4] Ethics Guidelines for Trustworthy AI, <https://ec.europa.eu/futurium/en/ai-alliance-consultation/guidelines>
Recent Comments